Over the last decades, computational social science has risen as a strongly empirical discipline, drawing on data science methods to tackle datasets that cannot be understood with simple analytical tools. This is particularly true in the study of public attention and news coverage: there are numerous studies looking at large-scale trends in Internet search queries and online petitions or applying natural language processing methods to news articles or social media activity. In other words, it is now possible to quantify, to a degree, what people talk about—anywhere from books and news to online social networks.
While some commentators have seen so-called “big data” as ending the need for theory, we argue these new data necessitate deeper theoretical understandings and better null model expectations. Human behaviour data are often compared against random baselines, but many social science research questions require more realistic, theory-informed null model expectations.
In particular for the social sciences, it is clear that the new models and theories we need to make sense of these new sources of large-scale data cannot rely on simple mathematical or statistical models alone, but rather need to incorporate knowledge from human behaviour. A good illustration of this difference between modelling natural and social behaviour is the study of how information spreads in social networks: while infectious diseases such as influenza might be captured by epidemiological models such as the SIR model, the transmission of information on social media can follow multiple different social mechanisms, some of which bear little resemblance to biological contagion.
Modelling the dynamics of public opinion
We presented ongoing work at the 5th International Conference on Computational Social Science, in Amsterdam, Netherlands, where we investigate how the effective number of issues holding public and media attention changes over time. Our data necessitate realistic, theory-informed models in order to ensure we answer our research question rather than report noise and artifacts in the data. To this end, we developed null models for the distribution of public attention over multiple issues. This is a relevant topic in the field of agenda setting, but also more broadly in the study of opinion dynamics and consensus formation. We investigate the diversity of issues within a public agenda, and compare the issue diversity in different data sources to a baseline expectation provided by our models.
Our data sources
We compared the behaviour predicted by our null models with data from public opinion polls and news media. For the former, we took data from British monthly attitudinal surveys collected by Ipsos MORI, who ask a representative sample of the UK population what they feel is the most important issue facing the country and code the responses into issue categories. The distribution of public attention over issues is not uniform: new issues are introduced over time, necessitating a realistic null model of our theoretical expectations for how public attention is distributed over an arbitrary number of issues.
Our second dataset consists of all articles from the German news magazine Der Spiegel, from 1947 to 2016. For this dataset, we looked at the patterns of language use over time using LDA topic modelling, and preliminary, manual inspection of the topics suggested they were typically related to issues in the policy agenda (e.g., defense, economy, environment).
Extracting the coverage dedicated to each topic in Der Spiegel over time allows us to study how the diversity of issues in a given dataset compares to an ensemble of random agendas of the same size. This can be calculated using the effective number of issues, which we use in a preprint of earlier work. When compared to an ensemble of random agendas, the public opinion polls show a lower effective number of issues at all points in time, indicating that despite the continuous shift of attention towards new issues, the total diversity of issues in the public agenda stays bounded.
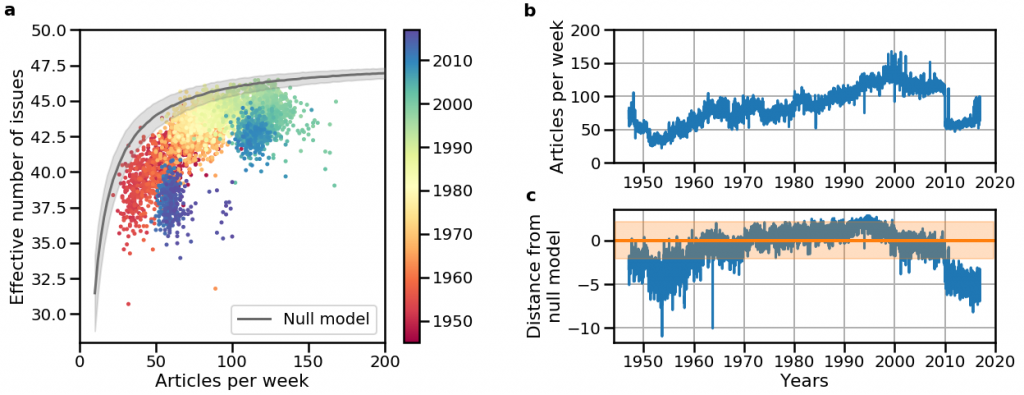
Panel (a) shows the number of articles per week in Der Spiegel, in comparison with the corresponding effective number of issues per week. In this panel, the color scale indicates publication year, and the grey line indicates the null model expectation (with one standard deviation) for the effective number of issues as a function of the number of articles. Panel (b) shows the number of articles per week decreases in 2010. However, the decrease in the effective number of issues by the news magazine appears to go beyond what would be expected. While the null model provides a close bound for most years, after 2010 the observed effective number of issues falls well below the null model expectation, which is shown with its 95% confidence interval in (c).
This analysis can also be performed when the number of issues is fixed, but the number of points composing each distribution of attention varies, which again requires a realistic expectation of how many issues we expect the news magazine to cover in a weekly edition with an arbitrary number of articles. This is the case of the news media data shown in panel (b) in the figure above. As the effective number of issues covered in a week might depend on the number of articles published, we compared every week with random samples of articles from Der Spiegel. The result is displayed in panel (a), which shows the effective number of issues observed in Der Spiegel stays below the null model prediction.
Panel (a) in the figure also shows that the magazine output drops after 2010. During the same period, its effective number of issues also moves further from the bound predicted by the null model, as shown in panel (c), suggesting this drop is not simply due to fewer articles, but indeed due to fewer issues covered by the news magazine. This resonates with the content of Der Spiegel at that time, as the financial crisis of 2008 led to increased coverage of economic matters, at the cost of other topics. Finally, beyond generating insights about policy agendas and public opinion, the null models we presented showcase how new models can provide useful insights to the study of how collective human behaviour. Our current work is further developing the null models to be able to understand how the rate at which agendas are observed (e.g., monthly vs. weekly) affects the data and, ultimately, to be able to control for this and other confounds when comparing different data sources.